The Distinct Advantages of Generative AI in Visual Art and Speech
Written on
Chapter 1: Understanding Generative AI's Strengths
Generative AI tools have demonstrated remarkable proficiency in creating visual art and audio, yet their writing capabilities often fall short, sounding mechanical and uninspired. This discrepancy can be traced back to the nature of the training data used for these models, as well as the historical context of art and language.
This paragraph will result in an indented block of text, typically used for quoting other text.
Section 1.1: The Influence of Historical Art
Rembrandt van Rijn, a luminary of the Dutch Golden Age, was born on July 15, 1606, in Leiden, Netherlands. Renowned as one of history's greatest painters, his legacy comprises over 300 paintings, 300 etchings, and 2,000 drawings, all characterized by the Baroque style of dramatic lighting and expressive detail.
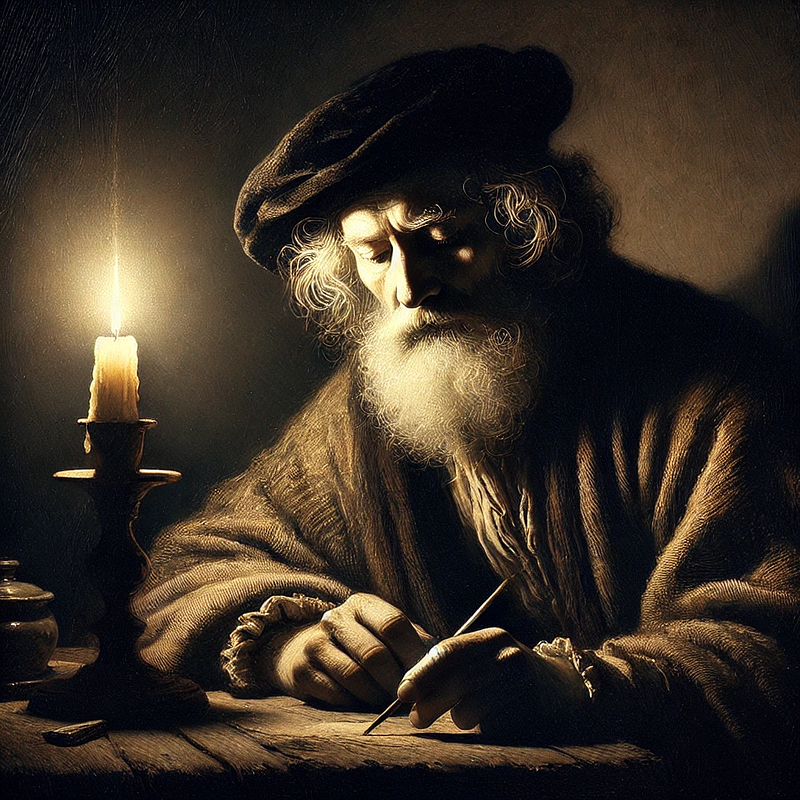
If a Dutch individual were to encounter Rembrandt today, they would likely struggle to comprehend his language. Despite his fluency in Latin, the vernacular of his time was Old Dutch, transitioning to Modern Dutch only after 1550. This evolution mirrors that of English, as William Shakespeare, another contemporary, wrote in a form of English that is not easily grasped by today's readers.
Section 1.2: The Challenge of Written Language
The primary reason generative AI excels in image generation over writing is the quality of its training data. While there are countless masterpieces from centuries of artistic history that AI can learn from, the same cannot be said for written content.
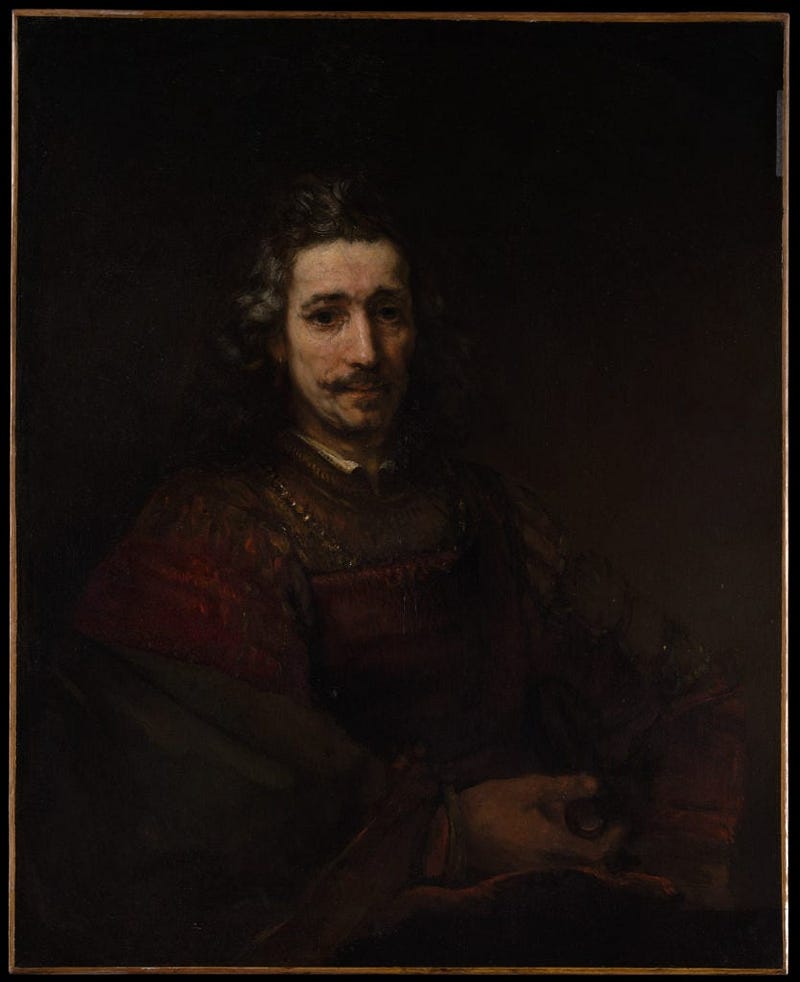
Throughout history, the pursuit of representational art has been paramount. Rembrandt's paintings continue to be celebrated not just as Baroque masterpieces but as significant contributions to the art world. In contrast, the vast majority of written content available today falls short of quality, with many writers lacking formal training in the craft. This results in a training dataset for language models that is often riddled with mediocre writing, reflecting a corporate tone rather than creative expression.
Chapter 2: The Nature of AI Training Data
When utilizing tools like ChatGPT, the output mirrors the style prevalent in corporate communication—simple, balanced, and devoid of depth. This is a direct consequence of the types of texts included in the training datasets, which primarily consist of online content since the dawn of the internet.
The first video titled Use of Generative AI Tools in Scientific Writing explores how these tools can enhance academic writing, showcasing the contrast between visual and written outputs.
The second video titled Generative AI for Academic Writing and Assessment: Issues and Opportunities discusses the potential and limitations of AI in the realm of academic writing.
Section 2.1: AI's Proficiency in Speech and Audio
The landscape of language processing is different; native speakers typically communicate effectively, which improves the quality of speech-to-text and text-to-speech applications. The training required for these functions is less demanding compared to the complexities of creative writing.
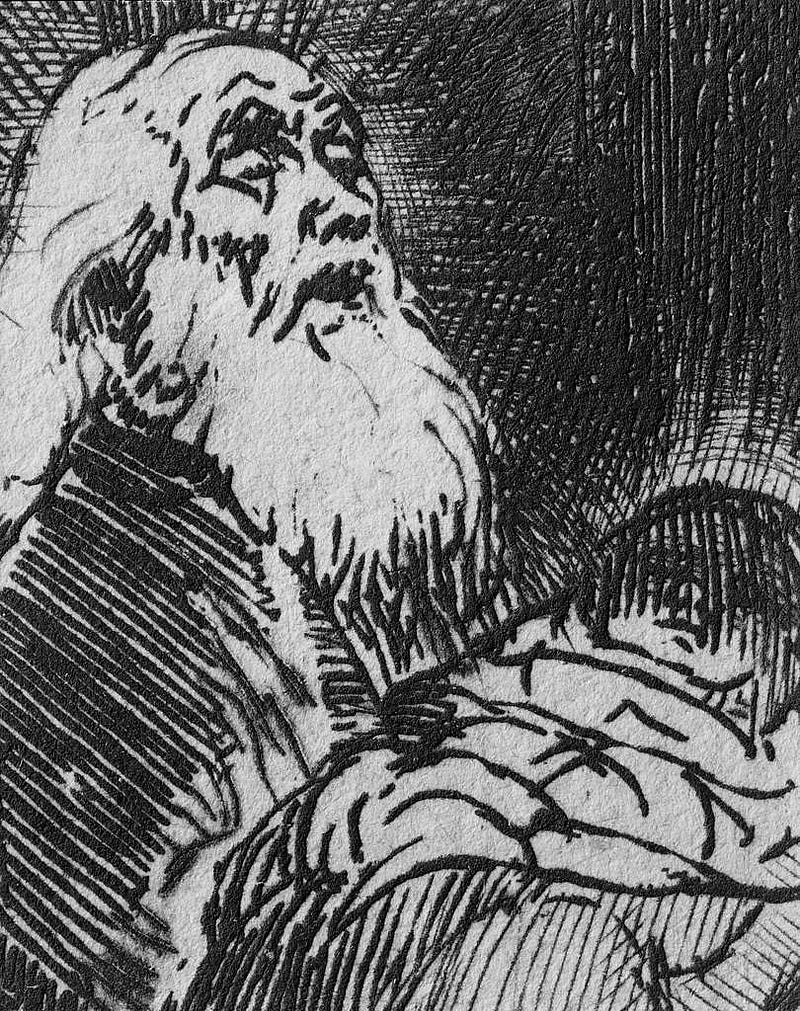
For instance, OpenAI Whisper was trained on an extensive dataset of multilingual audio, allowing it to achieve high accuracy in transcription tasks. In contrast, the vast array of written content includes a significant amount of subpar writing, diluting the quality of AI-generated text.
Section 2.2: The Limitations of Generative AI in Writing
Even though there is a wealth of written material available, much of it does not represent the pinnacle of literary quality. Tools like ChatGPT are trained on this entire spectrum, which includes low-quality texts, thus limiting their ability to produce compelling, original writing.
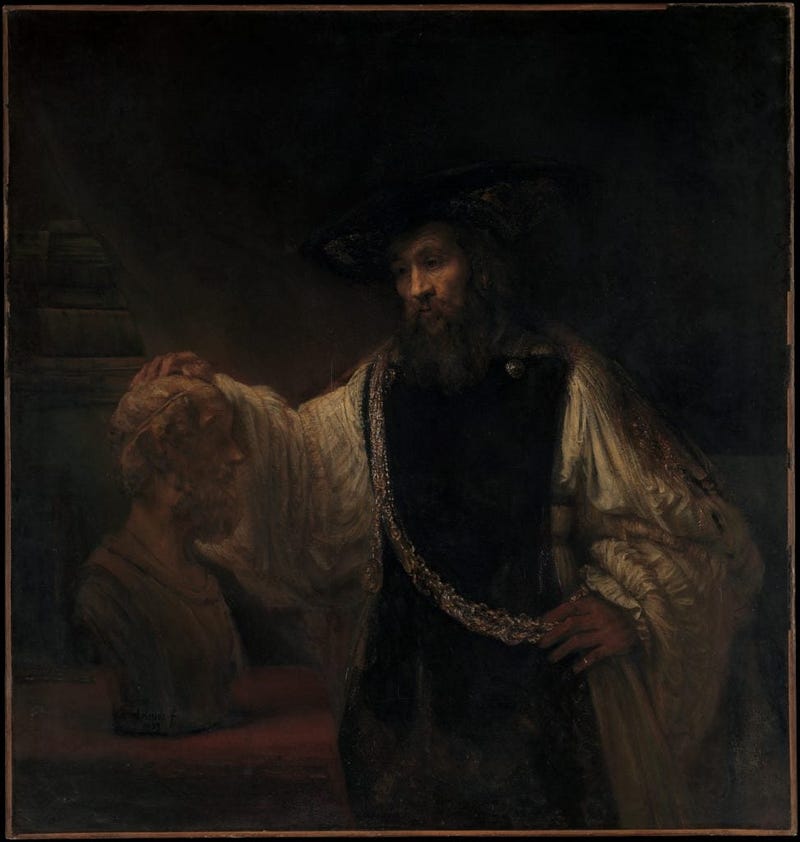
The same principle applies to the difference between visual art and written content. Artistic masterpieces have been preserved for centuries, while much of the writing available online is uninspired and formulaic. Consequently, generative AI excels in generating visual content but struggles with producing engaging written material.
Conclusion: Optimizing the Use of Generative AI
In summary, it is essential to recognize that while generative AI tools can yield impressive results in image and audio generation, their effectiveness in producing high-quality written content is limited by the training data's quality.
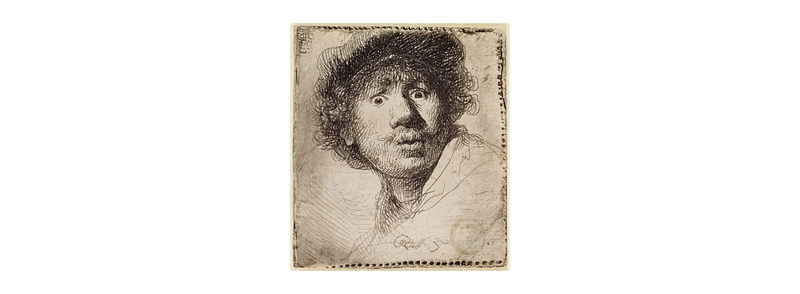
By understanding these distinctions, users can more effectively leverage these tools for tasks like transcription or generating realistic imagery rather than expecting the same level of quality in written outputs. This insight underscores the importance of input data quality in determining the effectiveness of AI-generated content.
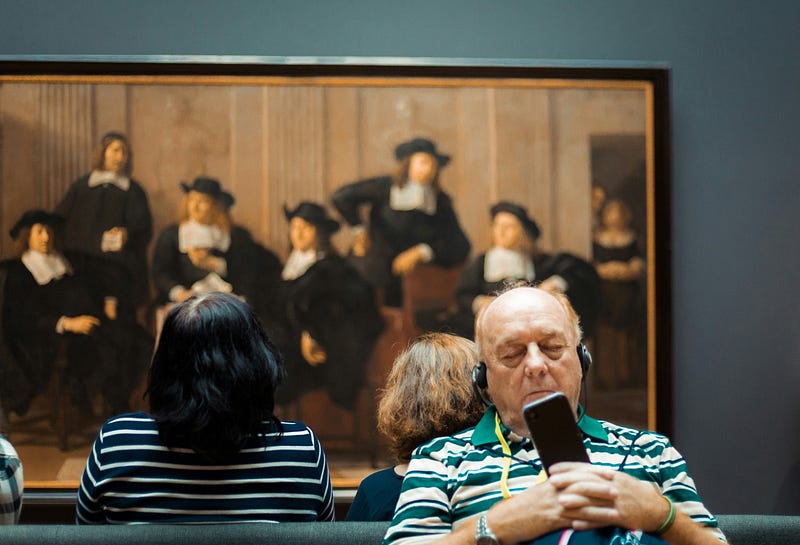
By acknowledging these limitations, users can make informed decisions about how to apply generative AI in their work, ensuring they maximize the benefits of this powerful technology.